Developing Digital Biomarkers (363-1163-00L)
This course is available during the fall semester at ETH Zurich. It gives an introduction to digital biomarkers and provides students with the foundations to develop their own digital biomarkers. More specifically, the course will cover fundamental topics such as designing observational studies, collecting, and exploring data generated by consumer-centric devices, and applying analytical methods to predict health-related outcomes.
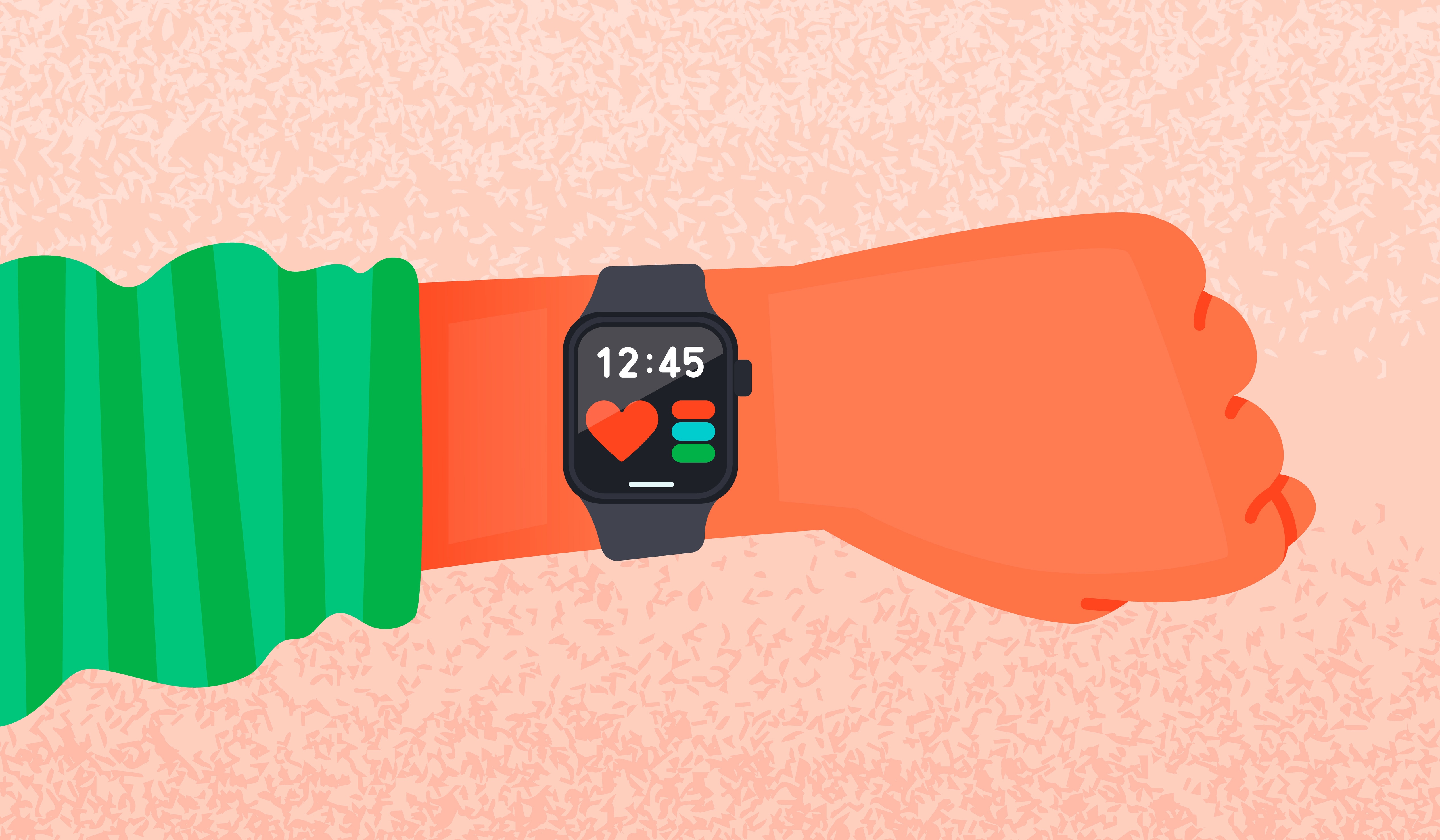
Course Information
Time: Thursdays, 2 p.m. – 4 p.m. (fall semester)
Location: CLA E4
Credits: 3 ECTS
Course Catalogue : 363-1163-00L
Lecturer: Dr. Filipe Barata
Teaching Assistants:
– Assignment 1: Patrick Langer
– Assignment 2: Fan Wu
– Assignment 3: Jinjoo Shim
Learning Goals and Objectives
The widespread use of mobile technologies (e.g., wearable sensors, mobile applications, social media, and location-tracking technologies) has the potential to meet the health monitoring needs of the world’s aging population and the ever-growing number of chronic patients. However, this premise is based on the application of Machine Learning algorithms that allow us to use this data in many different ways. In this course we will analyze systematic ways to collect data, review the most relevant methods and applications in healthcare, discuss the main challenges they present and apply the newly gained knowledge in practical assignments.
The course will consist of four topic clusters that will allow the discussion of the most relevant digital biomarker applications in healthcare:
-
Digital Biomarkers: From biological to digital biomarkers. How are they motivated, defined and how can they be leveraged for monitoring? Prognostic vs. diagnostic vs. predictive biomarkers. Passive sensing vs. active sensing. Digital biomarker vs. Digital therapeutics.
-
Consumer-centric device data: Today, vast amount of physiological, environmental, and behavioral observations can be collected with consumer centric devices. To derive clinical meaningful information from this data is, however, difficult. We will analyze strategies for extracting knowledge from those measurements.
-
Methodology: In the last decade, neural networks (also known as “deep learning”) have helped push the boundaries of the state-of-the-art in a myriad of domains. They have also uncovered a number of different problems. We will discuss advantages and disadvantage as well as alternative methods for their application to digital biomarker data.
-
Applications: Digital biomarkers are still an emerging subfield, but given that longitudinal in digital biomarker data are arguably easy to acquire in large quantities, it is expected that many relevant Machine Learning applications will emerge in the near future. We will review and discuss current applications and challenges.
After the course, students will be able to
- understand the anatomy of digital biomarkers
- understand the potential and applications of digital biomarkers
- be able to critically reflect and assess existing digital biomarkers
- be able to design and implement a digital biomarker